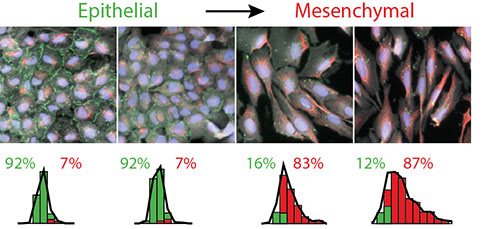
Brown researchers have trained a computer algorithm to spot a cellular transition associated with more aggressive cancers. Wong Lab / Brown University
PROVIDENCE, R.I. [Brown University] — Brown University researchers have developed a new image analysis technique to distinguish two key cancer cell types associated with tumor progression. The approach could help in pre-clinical screening of cancer drugs and shed light on a cellular metamorphosis that is associated with more malignant and drug-resistant cancers.
The epithelial-mesenchymal transition, or EMT, is a process by which more docile epithelial cells transform into more aggressive mesenchymal cells. Tumors with higher numbers of mesenchymal cells are often more malignant and more resistant to drug therapies. The new technique combines microscopic imaging with a machine learning algorithm to better identify and distinguish between the two cell types in laboratory samples.
“We know that there are these different cell types interacting within tumors and that therapeutics can target these cells differently,” said Susan Leggett, a doctoral student in Brown’s pathobiology graduate program and lead author of a paper describing the technique. “We’ve developed a model that can pick out these cell types automatically and in an unbiased way. We think this could help us better understand how these different cell types respond to drug treatment.”
The technique is described in an article published in Integrative Biology.
Generally speaking, the two cell types can be distinguishable by their shapes. Epithelial cells are more compact in appearance, while mesenchymal cells appear more elongated and spindly, both in their overall appearance and in the appearance of their nuclei.
“It’s not hard to distinguish the two in the most extreme instances,” said Ian Y. Wong, assistant professor of engineering at Brown and the senior author of the research. “But sometimes the shape differences are subtle and it can be hard for humans to recognize the difference, which makes categorizing the two a bit arbitrary. The innovation here is that we can train a computer to pick out those more subtle variations.”
That training was done by using an epithelial cell line, cultured in a petri dish, that serves as a model for human breast cancer. The researchers activated a transcription factor called Snail that is well known to cause these cells to quickly undergo an extreme form of EMT. Those cells, imaged before and after the transition, served as a training set to teach the algorithm to distinguish between the two cell types.
The researchers showed that, after training, the algorithm was able to categorize individual cells as either epithelial or mesenchymal with greater than 92 percent accuracy.
The team then used the algorithm to analyze sets of cells that undergo EMT triggered by pathways less well studied than that used in the training set. They treated epithelial cells with a compound called TGF-beta1, which promotes rapid cell growth and is also thought to induce EMT. They showed that the growth factor induced EMT more slowly than in the training set, and produced changes in cell shape that were subtler. Still, the algorithm was able to classify the cells after EMT with a high degree of confidence.
In a third experiment, the researchers looked at epithelial cells treated with the chemotherapy drug Taxol. Recent research has suggested that Taxol and other drugs, when delivered in sub-lethal doses, could induce EMT in the cells they fail to kill. In that way, the drugs may actually prime the tumors to become more drug resistant.
The experiment found that while sub-lethal Taxol created a range of cell shapes, more than 70 percent of those could be classified by the algorithm as mesenchymal.
It’s a preliminary finding that will require much more study to fully understand, Wong says. But it could shed light on how tumors become resistant to Taxol and other drugs.
“The acquisition of drug resistance and subsequent tumor relapse remains poorly understood,” Wong says. “This work suggests that EMT could be a mechanism through which tumors become more resistant.”
With more development, the researchers think their technique could provide a new means to screen the effectiveness of cancer drugs.
“When we do initial lab testing of drugs, we put cells on a plate, apply the drug and see what lives and what dies,” Wong said. “This could provide us with a more nuanced picture of the drug’s effects, and help us to see whether sub-lethal doses may prime cells for resistance.”
Wong says that another intriguing result from the study was that while the algorithm categorized most cells with high confidence, about 10 percent of cells in each of the experimental groups seemed to defy categorization. It’s possible, the researchers say, that this indicates an intermediate cell type that is somewhere between epithelial and mesenchymal.
“People have wondered if there might be more than two cell types of interest,” Wong said. “We can’t say for sure at this point, but we might be picking up a third type. That’s something we’ll be looking at in future work.”
Legget and Wong’s coauthors on the study were Jea Yun Sim, Jonathan Rubins, Zachary Neronha and Evelyn Kendall Williams, all from Brown. The research was supported by the National Institutes of Health (5T32ES007272-24), the COBRE Center for Cancer Research Development at Rhode Island Hospital (1P30GM110759-01A1), a Rhode Island Foundation Medical Research Grant, Jason and Donna McGraw Weiss and Brown University.