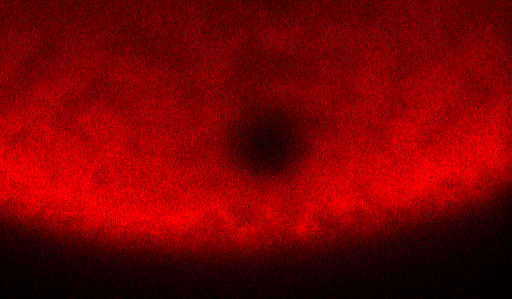
PROVIDENCE, R.I. [Brown University] — Understanding how proteins and other molecules move around inside cells is important for understanding how cells function. Scientists use an experiment called Fluorescence Recovery after Photobleaching, or FRAP, to investigate this molecular motion, and now Brown University researchers have developed a mathematical modeling technique that makes FRAP much more useful.
Traditionally, FRAP data have been used to measure molecular diffusion — the passive drifting of molecules within the jelly-like cytoplasm inside a cell. But these molecular movements aren’t always so passive. In many cellular processes, molecules can be transported actively by molecular motors, which tow molecules around like locomotives dragging lines of freight cars.
“We know that active transport is important in many cellular systems, but there wasn’t any way to capture it from FRAP data,” said Veronica Ciocanel, a Ph.D. student in Brown’s Division of Applied Mathematics. “We’ve developed a modeling technique for FRAP data that includes active transport and can quantify details about how those dynamics work.”
In a paper published in the Biophysical Journal, Ciocanel and her colleagues demonstrated the technique by describing new details about how egg cells redistribute genetic material before they begin dividing to form an embryo.
Getting more from FRAP
To perform a FRAP experiment, scientists tag molecules that they want to observe with glowing fluorescent proteins. Then they zap the area of interest with a laser, which deactivates some of the fluorescent proteins and creates a small dark spot within the glowing mass. Then scientists watch as the dark spot dissipates, which happens gradually as darkened molecules drift out of the spot and still-fluorescent molecules drift in. The amount of fluorescence in the spot as time progresses is what’s known as a recovery curve.
The recovery curve can then be fed into a mathematical model that generates a diffusion coefficient, an average rate at which the molecules drift around. Some models can also tease out a binding rate (the rate at which molecules stop moving by attaching themselves to some other molecule or substrate), but there weren’t any that could deal with active transport.
Ciocanel set out to create one in collaboration with a lab led by Kimberly Mowry, a professor of biology at Brown.
Active transport in egg cells
One of the things Mowry’s lab studies is RNA localization in egg cells, or oocytes. Before dividing to form embryos, oocytes redistribute messenger RNA — critical genetic molecules — from near the nucleus of the cell to the outer membrane on one of the cell’s sides. The process occurs across animal species and is essential to normal embryo development. Mowry’s lab studies it in a frog species called Xenopus laevis because the species’ oocytes are relatively large and easier to observe.
Mowry and other researchers had shown that active transport via molecular motors, along with diffusion, was likely important to the localization process in Xenopus oocytes. There was also speculation that the transport wasn’t unidirectional from the nucleus out to the membrane. Mowry had performed experiments suggesting that mRNA molecules actually move back toward the nucleus at times during the process. But it was impossible to capture all of those dynamics via FRAP.
Working with Björn Sandstede, chair of Brown’s Division of Applied Mathematics, Ciocanel developed models using sets of partial differential equations that could capture active dynamics. One model captured two states of molecular movement: simple diffusion as well as active transport in a single direction. A second more complex model captures diffusion, two-directional movement as well as the possibility that some molecules remain stationary for periods of time. Ciocanel then developed a set of numerical techniques to solve the model and give velocities for active transport motion.
Once the models were created and could be solved numerically, Ciocanel ran them on synthetic FRAP data from a hypothetical system in which the contributions from active transport were known. She showed that the models could correctly reproduce the active dynamics from the synthetic data.
Having validated the models, the researchers applied them to real data from FRAP experiments on Xenopus and were able to shed new light on the RNA localization process.
“We were able to quantify the contributions from each of the mechanisms,” Ciocanel said. “We can predict how much of the mRNA is diffusing, moving up and down or pausing along the way.”
The models were also able to confirm small but important nuances in the dynamics. For example, the research showed that bi-directional transport occurred more prominently in the part of the cell closest to the membrane.
New insights like these could ultimately help scientists to get a more complete picture of the dynamics at play in this critical cellular process. But this is far from the only setting where the technique could be helpful. Active transport is known to occur in many cellular processes. Synaptic activity in the brain, for example, is thought to involved active mRNA localization.
“Whenever there’s active transport,” Sandstede said, “this method allows you to learn about what’s happening.”
The research was supported by the National Science Foundation (DMS-1408742) and the National Institutes of Health (GM071049).