PROVIDENCE, R.I. [Brown University] — The past few years have witnessed a revolution in artificial intelligence. AI systems are beating humans on reading comprehension tests, clobbering board game champions and enabling cars to drive themselves. Even more mundane AI systems, like smartphone apps that recognize faces and personal assistants that understand verbal commands, were seemingly insurmountable challenges just a decades or so ago.
These recent breakthroughs have been made possible in large part by a technology known as deep learning or deep neural networks — algorithms that have become the unseen force behind modern AI. Every time a phone responds to “Hey Siri,” or Google translates a sentence from Swedish to Swahili, deep neural networks are at play.
But for all the success of deep learning, the algorithms have their weaknesses, and there remains a yawning chasm between what human intelligence is capable of and what machines can do. This week at Brown, researchers from computer science, mathematics, biology and psychology will gather discuss how deep learning falls short and what neural principles might power the next AI revolution.
The event is the first in a symposium series dubbed Beyond Deep Learning, which will feature keynote talks from renowned experts as well as breakout sessions led by Brown faculty from disparate disciplines. The keynotes, which take place on Jan. 18 and 19 at 2 p.m. in Metcalf Auditorium, are free and open to the public. Speakers include Mathias Bethge from the Max Planck Institute for Biological Cybernetics, Gary Marcus from New York University, Sam Gershman from Harvard University and Randy Gallistel from Rutgers University, along with Brown faculty members Stephanie Jones, Michael Frank and George Konidaris.
Thomas Serre, an associate professor in Brown’s Department of Cognitive, Linguistic and Psychological Sciences, is one of the organizers of the conference, which is supported by Brown’s Center for Vision Research, Humanity Centered Robotics Initiative, Brown Institute for Brain Science, Brown Media Services and the Computation in Brain and Mind Initiative. Serre discussed the conference series in an interview.
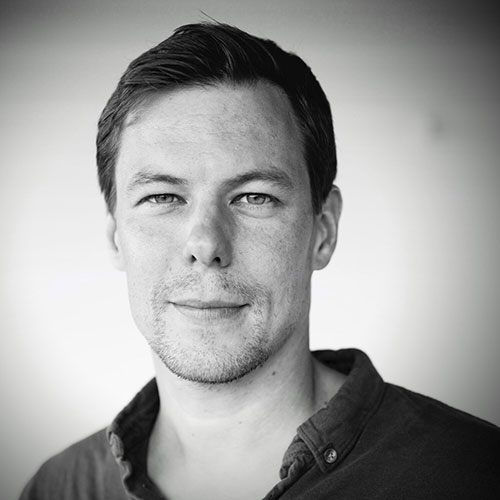
Q: Could you explain a bit about what deep learning is?
A: Deep learning and deep neural networks describe a general class of algorithms that is pushing the state of the art in every area of artificial intelligence. These algorithms are roughly inspired by the networks of neurons in the brain that comprise the visual system. The “deep” in deep learning refers to how many layers of artificial neurons there are in the network. Old-school neural networks, which have been around for many years, had a handful of layers of processing. But today we have networks with more than dozens if not hundreds of layers of processing, which has made them much more powerful.
These algorithms are great at learning things from training. If you want a system that can discriminate cats from dogs, we feed a deep learning algorithm lots of images labeled “cat” and lots of images labeled “dog.” By learning what it is about the images that’s specific to either cats or dogs, the algorithm can make rules to reliably categorize those two things.
These algorithms are absolutely everywhere in AI. They’re what’s behind AlphaGo, Alexa, Siri, self-driving cars and most other cutting-edge AI technologies.
Q: If it has been so successful, then why host a conference to think about what might replace it?
A: Deep learning has been responsible for some really major breakthroughs; there’s no doubt about that. But there’s also, I think, some over-hype going on. While we celebrate the achievements, the limitations in what deep learning can do are swept under the rug a bit.
One shortcoming deals with the robustness of these systems. In many cases, it’s not particularly hard to confuse them. One example would be some recent research on the system Google uses for self-driving cars. Researchers have shown that stickers with particular patterns can be put on traffic signs that completely confuse the system. You could have a stop sign, for example, with a sticker placed on it that makes the system think it’s a 65-mile-per-hour speed limit sign. That could be a real problem. There are also whole classes of problems that deep learning isn’t very good at solving. For example, work in my lab has shown that deep neural networks have trouble with spatial relations — figuring out if one object is to the left or right of another, for example.
So the idea for the conference is to bring researchers from psychology, cognitive science and neuroscience together with researchers from computer science and mathematics. We want to see if we can leverage that combined expertise to work on some of the limitations of deep learning and start to think about how these algorithms could be improved or replaced.
Q: Are there ideas out there about what the next-generation AI might entail?
A: It’s really wide open still, but there are interesting avenues emerging.
We know from work in computational neuroscience that there’s something about deep neural networks that’s consistent with how people perform basic visual recognition tasks. But work from my lab and others has shown that deep learning only approximates the first few-hundred milliseconds of the visual processing that happens in our heads. In other words, when we force people to solve visual tasks very quickly, we find that the human responses correlate very well with the algorithms, meaning we get similar correct and incorrect responses. But when we give people more time to perform the tasks, they start improving vastly over the algorithms. So the question is: What’s happening in our human brains that enables us to overcome the challenges that confuse deep neural networks?
That’s where biology and neuroscience can inform us. You can think of this workshop as laying out some of the additional mechanisms underlying biological vision, and then thinking about whether it would make sense to try putting these things into modern AI architectures.
Q: Obviously, it’s not possible to solve these questions in one conference, but what do you hope to achieve?
A: In my own experience, I’ve found that it’s not always easy to get my psychology and neuroscience colleagues to interact with my computer science and engineering colleagues. So we want to bring people together in a relaxed atmosphere where they can start exchanging ideas without worrying about saying something wrong. We want to get people speaking the same language and lay the groundwork for collaborations.
We also hope this is a step toward building our computational neuroscience community here at Brown. Together with colleagues, we launched the Initiative in Computation in Brain and Mind, which is one of the sponsors or this event. We have first-class researchers in this area and we want to nurture an ecosystem where students in applied math, engineering, computer science, neuroscience and cognitive sciences are able to talk to each other with the goal of building new ideas and answering big questions.
We really see Brown as a potential leader in this area, and this series is one thing we can do to cultivate that. I'd like to thank graduate student Matt Ricci and postdoctoral researcher Drew Linsley for all their work in helping to put this workshop together.