Virtual Communities, Virtual Families
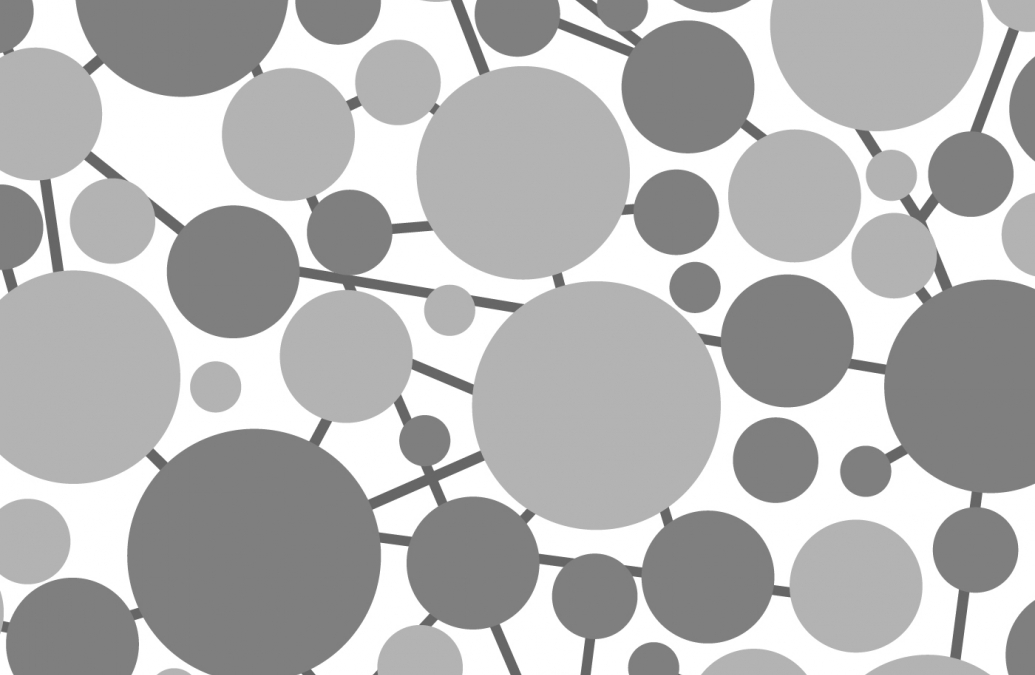
Mara Freilich '15 is a Royce Fellow conducting research on the effectiveness of current methods of incorporating phylogenetic information into ecological diversity metrics to infer community assembly processes.
Tropical North Queensland, where the Daintree Rainforest meets the Great Barrier Reef. Using phylogenetic trees might help understand why these two areas have so much biodiversity.
Are ecological communities just random collections of species? If not, what determines which species can live where? Does the environment select which species can survive or do species compete with each other for shared resources? Even more pressing, how are ecosystems changing in response to overfishing, climate change, and introduced species? Increasingly, we are learning that these threats not only endanger species survival, but can also alter community assembly.
Part of the reason ecologists can only tentatively answer these questions is a lack of tools to measure and analyze community assembly processes. Hypothetically, we could measure the way every species interacts with its environment and the other species in its community. But simply putting on a snorkel mask and glimpsing the awe-inspiring biodiversity of the Great Barrier Reef is enough to convince anyone that this approach is impossibly time consuming. Instead, researchers are asking what known information can we use as a proxy for unknown information.
I'm studying a new approach based on a paradox set forth by Charles Darwin; closely related species compete more strongly because they share more resources, but they will also be more suited for the same environments, again because they use the same resources. The idea is to use the relatedness of a community as a substitute for unknown information about species interactions. If a community is less related than expected by chance, we might be able to infer that the community is structured by competition. But if the community is more related than expected by chance, we might say the environment selects which species can live where. For example, microbes in rivers with heavy metals are more related than expected by chance, because only a few are adapted to that extreme environment. Applications of this approach are still being innovated, but one relevant example is the creation of marine reserves. If we have the goal of protecting biodiversity by preserving ecosystems, we have to understand which aspects of communities (do we protect diverse environments or critical species?) are more important to protect.
Since this approach is very new it needs to be tested and refined. We know the use of proxies can only go so far, the questions are, how far and will they ever give misleading results? We test new tools using model data from well-understood natural systems or well-understood mathematical models. I am simulating data, making virtual species, with simulated relationships to other species, and assembling them into virtual ecological communities using very simple models to test how well the approach of measuring relatedness works under different assumptions. The number of samples I have generated this summer, 2.5 million, is overshadowed by the total biodiversity on Earth, 8.7 million eukaryotic species at the last estimate.
Given that great potential for species interactions, my first challenge was to decide which model to use and which assumptions to include. Next, given the great number of results, was interpreting the model outcomes in terms of ecology. At the end of the day, it's a constant push and pull between reality and models, whether the models are expressed in words or equations. Modelers need to spend time appreciating the complexity of natural systems, but at the same time the modeler's continual focus on which assumptions are being made is extremely useful in crafting elegant explanations for complex processes.